Science is about finding explanations. With the abundance of data, we have stopped asking for explanations and are satisfied with mere correlations. We are happy to know something will happen without knowing why it will happen. This is damaging the spirit of scientific enquiry in a fundamental way from which we may never recover.
The scientific method has guided the development of science over the last four hundred years. The method involves carefully recording observations, formulating a meaningful hypothesis, and rigorously testing the hypothesis. Based on the outcomes of these tests, the hypothesis is refined over and over again till it can explain the observations. Scientists are taught correlation does not imply causation. For them, it is not enough to know that something happens; they need to understand the underlying mechanisms that cause it to happen.
Artificial intelligence (AI) is a very different beast. Most AI systems use a technique called machine learning to teach computers how to perform tasks by providing them large datasets relevant to the task. The developers that build such data-driven AI systems are called data scientists. Data scientists, unlike real scientists, are satisfied with correlation and are not concerned with causation. They flout the principles of the scientific method and scoff at its rigour. They blatantly ignore the need for a theory and are interested only in empirical models that spit out predictions without any understanding of what they are predicting.
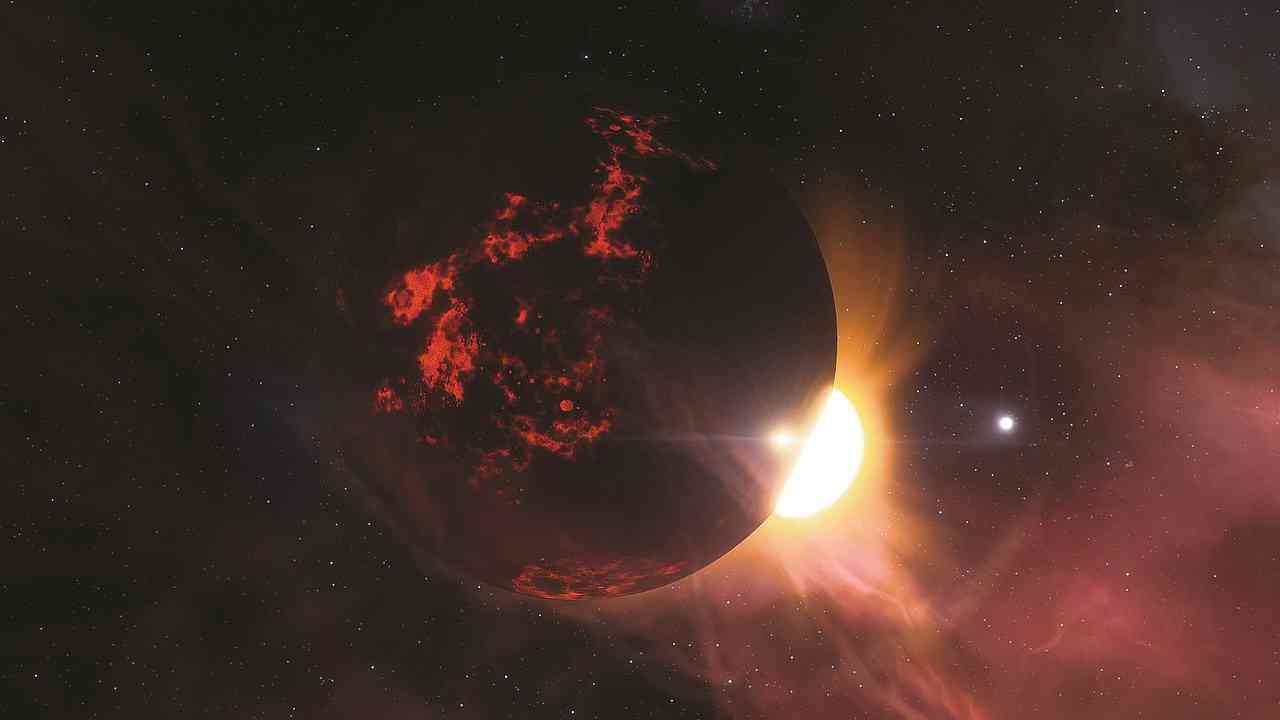
The Three Body Problem is a well-studied problem in physics that was first described by Sir Isaac Newton in his immortal treatise Principia Mathematica. Suppose you are given three large objects in space — say the Sun, Moon, and Earth — and their initial locations and velocities, can you determine their final locations after a fixed time interval? To solve the problem, you need to calculate the effects of the gravitational force each body exerts on the other two bodies. This might sound simple, but this problem has baffled physicists for centuries. No one has been able to derive the equations that provide a final solution. Computer simulations are used for the task which laboriously compute the motion of each body at every time-step using the laws of motion. These calculations are chaotic i.e. very sensitive to small changes. Due to this chaotic nature and the massive number of computations involved, the simulations take weeks or months to run, can get stuck, and are sometimes simply unfeasible.
In October 2019, AI researchers announced that they developed a neural network that could find accurate solutions to the Three Body Problem in a matter of seconds. This is a staggering 100 million times faster than today’s best simulations. Neural networks are the most popular machine learning algorithms, and they form the heart of most AI solutions. What makes them immensely powerful is their ability to be universal approximators. Given enough training data, they can learn to closely approximate any equation that describes the relationship between inputs and outputs. In the above case, the researchers trained their neural network on a dataset of 10,000 three-body configurations. The neural network processed this dataset and learned the equation that describes the relationship between the initial configuration and the final configuration without knowing anything about the laws of motion.
AI is making rapid inroads in physics, chemistry, biology, finance, economics and even social sciences where it is quickly replacing traditional theoretical approaches. In 1973, Fisher Black, Myron Scholes, and Robert Merton developed the famous and highly influential Black-Scholes formula for pricing stock options for which Merton and Scholes were awarded the Nobel Prize in Economics. Their approach was inspired by Brownian Motion that physicists use to model the movement of particles suspended in a fluid. In the last decade, the theory-driven Black-Scholes model has been practically rendered obsolete by data-driven neural networks that learn to predict future prices only from past prices without understanding a thing about financial markets.
In healthcare, AI models are routinely employed to diagnose conditions like tuberculosis and various cancers from medical images. Again, the AI knows nothing about radiology, medicine, or human anatomy.
Science is about finding explanations. With the abundance of data, we have stopped asking for explanations and are satisfied with mere correlations. We are happy to know something will happen without knowing why it will happen. This is damaging the spirit of scientific enquiry in a fundamental way from which we may never recover.
Scientific thought is a skill that we, as a society, did not develop overnight. It took us millennia to discover it and then centuries to adopt and start practising it. Like we are losing the abilities to commit things to memory, do arithmetic in our head, or navigate the streets without GPS, how long will it be before we add scientific thought to this fast-growing list of abilities technology has replaced?
If you liked the article, follow me on LinkedIn or Twitter, view my personal webpage, or email me at viraj@berkeley.edu.
Write a comment ...